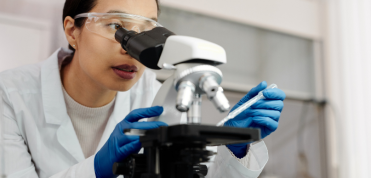
Imagine a world without animal testing… where the safety of chemicals, cosmetics, therapeutics and other products is assured using modern, human biology-based predictive tools. Making this world a reality is the goal of the AFSA Collaboration.
Imagine a world without animal testing… where the safety of chemicals, cosmetics, therapeutics and other products is assured using modern, human biology-based predictive tools. Making this world a reality is the goal of the AFSA Collaboration.
READ MORE
“I predict that 10 years from now, safety testing for newly developed drugs…will be largely carried out using human biochips…This approach…will mostly replace animal testing for drug toxicity and environmental sensing, giving results that are more accurate, at lower cost and with higher throughput.”
Francis Collins, MD, PhDThe Animal-Free Safety Assessment (AFSA) Collaboration is a unique multi-sector partnership between non-profit, corporate, and philanthropic leaders working to advance acceptance and use of animal-free safety science as a gold standard across regulatory frameworks worldwide. Meet our members:
The Animal-Free Safety Assessment (AFSA) Collaboration brings together corporate and non-profit leaders who share the goal of accelerating a modern, species-relevant approach to safety assessment globally to better protect people and our planet, and hasten the replacement of animal testing. Meet our members: